Synthetic Data Is a Dangerous Teacher
Synthetic Data Is a Dangerous Teacher
Synthetic data is data that is artificially created rather than being generated by real-world events. While synthetic data can be…
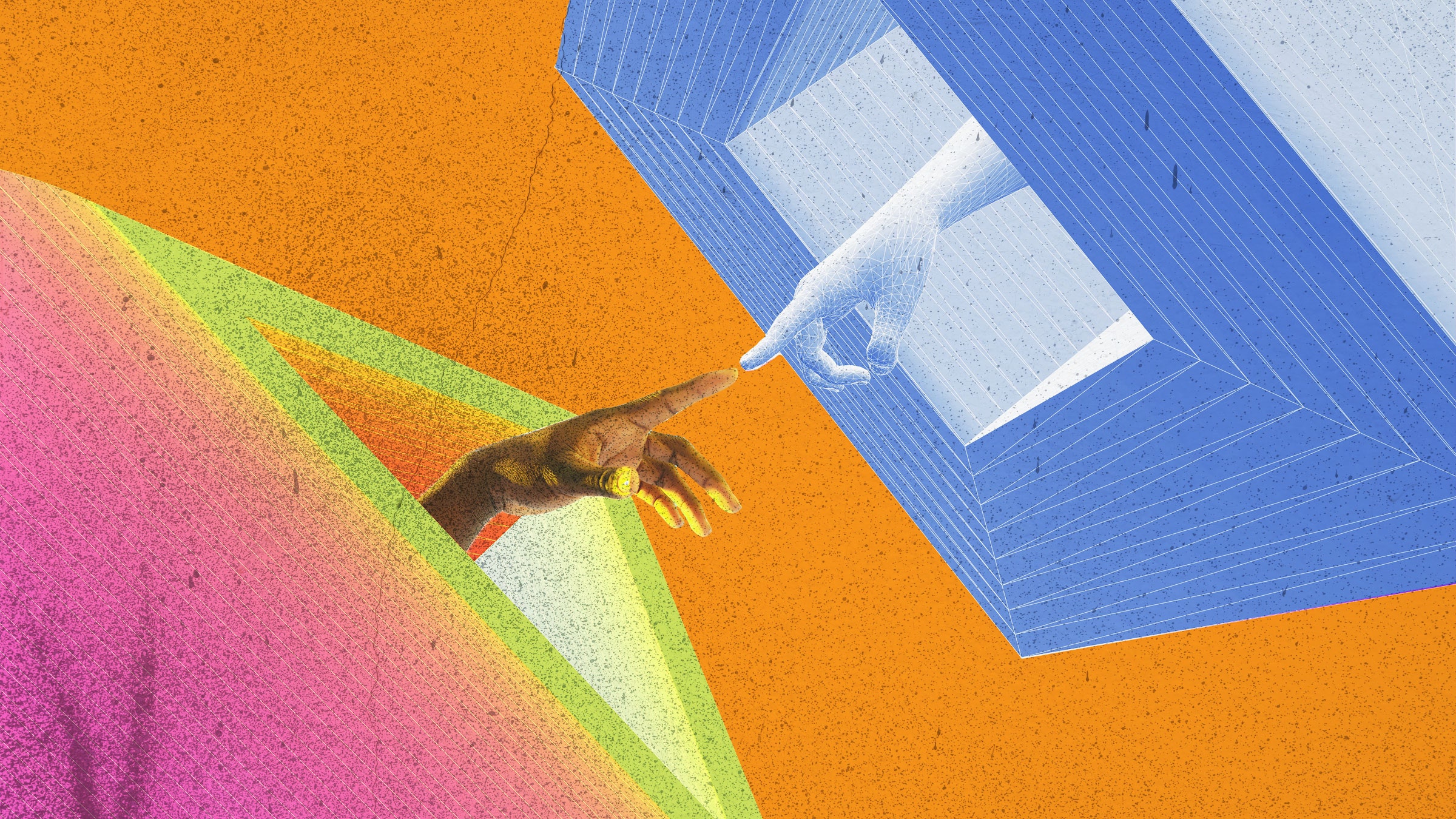
Synthetic Data Is a Dangerous Teacher
Synthetic data is data that is artificially created rather than being generated by real-world events. While synthetic data can be useful for testing and training machine learning models, relying too heavily on it can be dangerous.
One of the main dangers of synthetic data is that it may not accurately reflect real-world scenarios. This can lead to models that are not effective when deployed in the real world.
Another danger of synthetic data is that it can perpetuate biases and stereotypes that exist in society. If synthetic data is based on biased or flawed assumptions, it can reinforce those biases in machine learning algorithms.
Additionally, synthetic data may not capture the complexity and nuance of real-world data. This can lead to models that are overly simplistic and fail to capture the full range of variability in the real world.
It is important for data scientists and machine learning practitioners to use a combination of real and synthetic data in their training and testing processes. This can help ensure that models are robust and effective when deployed in real-world scenarios.
By being aware of the dangers of synthetic data and taking steps to mitigate them, we can ensure that machine learning algorithms are fair, unbiased, and effective.
In conclusion, while synthetic data can be a useful tool for training machine learning models, it is important to be cautious and aware of its limitations. Using a balanced approach that incorporates both real and synthetic data can help ensure the reliability and accuracy of machine learning algorithms.